Part One: The Need for Equity Approaches in Quantitative Analysis
by Lois Joy, Ph.D., Research Director for Jobs for the Future, and member of the CTE Research Network Equity Working Group.
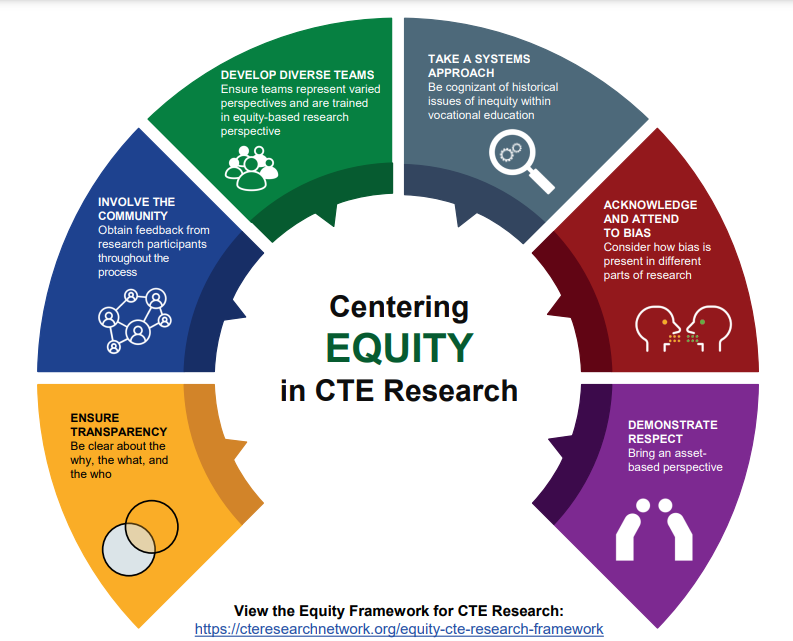
To support equity in CTE research, the Equity Working Group from the Career and Technical Education Research Network (CTERN) authored an Equity Framework for CTE Research which provides principles and practices for researchers on equity questions, designs, and implications throughout the research process. While this guide was authored with a specific focus on Career and Technical Education (CTE) research, the ideas within represent the expertise of a variety of experts in education research. Researchers from all social sciences could implement most if not all the recommendations. This part one of a two-part blog that focuses on quantitative data analysis with part one commenting on the need for equity considerations and approaches in quantitative data analysis and part two recommending practices and approaches that support equity.
For the quantitative analysis portion of the CTE Equity Framework, we drew from the emerging fields of quantitative criticalism, QuantCrit, and Critical Quantitative analysis to explore the need for and approaches to an equity approach to quantitative data analysis. These approaches emerged in recent years in a portfolio of thought that examines the impacts of structural racism and sexism on quantitative data analysis and policy recommendations in education and other fields including public health, sociology, and economics. New scholarship has begun to explore the nuances and differences in these approaches and their philosophical lineages which includes critical race theory, Black feminism, conflict theory, class struggle, and feminist economics.1 Our approach in the CTE Framework was to draw high level insights from this body of work to inform equity in CTE data analysis that can apply to groups of people who may face systemic barriers to CTE participation including, for example, Black and Hispanic learners, people who identify as women and nonbinary, and people with disabilities.
Learn more about using the Equity Framework in your research!
Critical quantitative theories have brought to our attention how quantitative analysis is not without embedded biases. Bias here refers to the way in which preconceived assumptions about people of different races, ethnicities, and genders, made consciously or unconsciously, can become embedded into data analysis leading to findings and policies that perpetuate rather than alleviate structural barriers to access, advocacy, and resources for success or advancement. These biases creep into all aspects of data analysis, from coding and cleaning of data to estimating and interpreting the findings. The goal of an equity lens is to build awareness of these biases in data analytics and the development of tools and practices to reduce the bias. A first step for researchers interested in reducing social, education, and economic injustice rather than reproducing structural inequalities is uncovering the sources of these biases in all aspects of data analysis.
At its root, quantitative data analysis consists of categorizing inputs and outputs into relevant groupings and drawing connections between them. None of this is self-evident from the data itself but subject to the assumptions and hypotheses that individual researchers bring to the analysis. The data don’t objectively “speak for themselves” but are shaped, merged, and connected by researchers who make many decisions along the way about how to do this. Systematically interrogating assumptions in our data analysis will help us to uncover our own blind spots.
A key insight of Critical Quantitative Theories and related scholarship is that categories of gender, race/ethnicity, and other socially constructed demographic groupings are not only social identities but also capture the roles of structural systems of oppression on outcomes, which are hard to observe and tease out of limited datasets. More specifically, coefficients on genders, race/ethnicity, and other demographic categories represent the impact of sexism, racism, and other “isms” that emerge from social, educational, and economic processes and power dynamics.3 “ Controlling for” differences that are associated with demographic variation may, in fact, mean modeling away (and thus losing sight of) the impacts of those “isms.”
Making the structures of oppression visible is the challenge for quantitative researchers (the subject of the Part 2 blog on this topic). When this is not possible, researchers must be transparent about the limitations of the analysis. This approach to making structural barriers more visible in the analysis also will help address what has historically been a bias toward “deficit approaches” in education research. Deficit approaches blame learners for educational and outcome gaps, and the impacts of systemic barriers remain unexamined. 4 To more accurately capture the lived experiences of individuals impacted by sexism, racism, and other forms of discrimination, researchers should use strategies to minimize biases and clearly articulate the limitations of quantitative analysis. Mixed-methods approaches which include qualitative data on these lived experiences can be considered as a key strategy to overcome these limitations.
This is the fifth in an eight-part blog series on the Equity Framework for Career and Technical Education Research authored collaboratively by the CTE Research Network’s Equity Working Group and previously published by the American Institutes for Research.
More Methodspace Posts about the Equity and Research
This blog is the seventh, and penultimate post, in a follow-on to our 2021 “The future of computational social science is Black” series, about a Summer Institute in Computational Social Science organized by Howard University and Mathematica. It continues to bring the power of computational social science to the issues of systemic racism and inequality in America. This marks the third iteration of the successful SICSS model being hosted by a Historically Black College or University.
This blog post is the sixth of eight in a follow-on to our 2021 “The future of computational social science is Black” series, about a Summer Institute in Computational Social Science organized by Howard University and Mathematica. It continues to bring the power of computational social science to the issues of systemic racism and inequality in America. This marks the third iteration of the successful SICSS model being hosted by a Historically Black College or University.
Latanya Sweeney, scholar of technology science, Daniel Paul Professor of the Practice of Government and Technology at the Harvard Kennedy School and in the Harvard Faculty of Arts and Sciences, and director and founder of the Public Interest Tech Lab, delivered the keynote address for SICSS-Howard/Mathematica 2023.
This blog post is the fifth of eight in a follow-on to our 2021 “The future of computational social science is Black” series, about a Summer Institute in Computational Social Science organized by Howard University and Mathematica. It continues to bring the power of computational social science to the issues of systemic racism and inequality in America. This marks the third iteration of the successful SICSS model being hosted by a Historically Black College or University.
This blog post is the fourth of eight in a follow-on to our 2021 “The future of computational social science is Black” series, about a Summer Institute in Computational Social Science organized by Howard University and Mathematica. It continues to bring the power of computational social science to the issues of systemic racism and inequality in America. This marks the third iteration of the successful SICSS model being hosted by a Historically Black College or University.
This blog post is the third of eight in a follow-on to our 2021 “The future of computational social science is Black” series, about a Summer Institute in Computational Social Science organized by Howard University and Mathematica. It continues to bring the power of computational social science to the issues of systemic racism and inequality in America. This marks the third iteration of the successful SICSS model being hosted by a Historically Black College or University.
This blog post is the second of eight in a follow-on to our 2021 “The future of computational social science is Black” series, about a Summer Institute in Computational Social Science organized by Howard University and Mathematica. It continues to bring the power of computational social science to the issues of systemic racism and inequality in America. This marks the third iteration of the successful SICSS model being hosted by a Historically Black College or University.
This blog post is the first of eight in a follow-on to our “The future of computational social science is Black” series, about a Summer Institute in Computational Social Science organized by Howard University and Mathematica. It continues to bring the power of computational social science to the issues of systemic racism and inequality in America. This marks the third iteration of the successful SICSS model being hosted by a Historically Black College or University.
Applying an equity focused lens specifically to reporting and dissemination necessitates a careful and deliberate approach. Learn more in this post!
Learn about disseminating research with an equity lens in this guest post from the CTE Research Network Equity Working Group.
Given the difficulties that emerged with the global Covid pandemic, the European Commission funded the PREPARED project. The aim of the project is to develop an ethics and integrity framework to guide researchers working to prevent and address large-scale crises. Find meeting reports, recordings, and related posts.
The Career and Technical Education (CTE) Equity Framework approach draws high-level insights from this body of work to inform equity in data analysis that can apply to groups of people who may face systemic barriers to CTE participation. Learn more in this two-part post!
The Career and Technical Education (CTE) Equity Framework approach draws high-level insights from this body of work to inform equity in data analysis that can apply to groups of people who may face systemic barriers to CTE participation. This is part 2, find the link to part 1 and previous posts about the Equity Framework.
Some of us feel that technology is everywhere, but that is not the case for everyone. Inequalities persist. What do these disparities mean for researchers?
Why does identity matter in the methods classroom?
How to look at data collection using an Equity Framework for CTE Research, which provides principles and practices for researchers on equity questions, designs, and implications throughout the research process.
Find a multidisciplinary collection of scholarly and historical articles about Juneteenth.
The opening plenary of SICSS-Howard/Mathematica 2022 featured a fireside chat with Dr. Anthony K. Wutoh, the Provost of Howard University, and Dr. Amy Yeboah Quarkume, an Associate Professor of Africana Studies, to kick off the event.
The first Summer Institute in Computational Social Science held at a Historically Black College or University, returns to Howard University for its two-day pre-institute, Praxis to Power for graduate students, postdoctoral researchers, and beginning faculty who needed more time to practice computational methods.
For researchers interested in incorporating equity into their work, it all starts at the very beginning with designing the study. Learn more in this guest post!
The Equity Working Group of the CTE Research Network (CTERN) has published a new framework for any researcher in social or education fields on using an equity lens to do their research. Find a link to The Equity Framework for CTE Research and information about a free webinar.
Summer Institute in Computational Social Science site sponsored by Howard University and Mathematica (SICSS-Howard/Mathematica) awards individuals and teams for the inaugural Excellence in Computational Social Science Research Fund as a unique and exclusive benefit offered to alumni of the site.
A SICSS-Howard Mathematica 2021 participant shares how he reconnected with others in a meaningful way and grew personally during his virtual SICSS experience.
Paul Decker PhD, president and chief executive officer of Mathematica and nationally recognized expert on policy research, delivered the closing plenary address on Friday, June 25th at SICSS-Howard/Mathematica 2021.
Timnit Gebru, co-founder of Black in AI, advocate for diversity in the field of technology, and Fortune’s Top 50 Leaders in the World in 2021 delivered the keynote address for SICSS-Howard/Mathematica 2021.
The first Summer Institute in Computational Social Science hosted at a Historically Black College or University featured a panel of guest speakers who inspired participants with their research and professional trajectory. Lecture topics include re-entry into the job force for incarcerated people, financial statuses of small businesses in relation to the COVID-19 pandemic, social identities and systems of power, and discriminatory bias within technology.
SICSS-Howard/Mathematica participants had the benefit of novel Bite-Sized Lunchtime Talks during the inaugural SICSS at a Historically Black College or University. The purpose of this SICSS-H/M specific site innovation was to introduce participants to people and organizations doing impactful and complementary work with data.
Wayne A.I. Frederick, seventeenth president of Howard University, delivered the opening plenary address on Sunday, June 13th at the end of SICSS-Howard/Mathematica 2021’s pre-institute, Praxis to Power.
This blog post is the second of nine in a series called “The Future of Computational Social Science is Black” about SICSS-Howard/Mathematica 2021, the first Summer Institute in Computational Social Science held at a Historically Black College or University.
This blog post is the eighth, and final, post in a follow-on to our 2021 “The future of computational social science is Black” series, about a Summer Institute in Computational Social Science organized by Howard University and Mathematica. It continues to bring the power of computational social science to the issues of systemic racism and inequality in America. This marks the third iteration of the successful SICSS model being hosted by a Historically Black College or University.