What is randomness?
by Stephen Gorard, PhD. Dr. Gorard is Professor of Education and Public Policy, and Director of the Evidence Centre for Education, at Durham University. He is the author of How to Make Sense of Statistics, and served as a Methodspace Mentor in Residence in 2021.
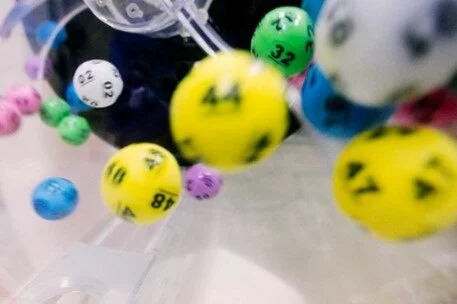
In statistics and the philosophy of science, “randomness” means something rather more than its everyday meaning of merely haphazard, or without apparent pattern. It is stronger, in the sense that something either is or is not random. There are no degrees of randomness, because a random event is one that is completely unpredictable in form, outcome or timing. Randomness is the quality of such an event – its unpredictability, and lack of intention.
Applied to a set of numbers, randomness means that each number in the set is unrelated to any other. Knowing one or more numbers in the set will not help identify any other numbers in the set. By analogy, if a standard six-sided die roll has a random outcome, then knowing the previous 10, 100 or 1,000 results from that die will not assist you in predicting of the next one. The chances of guessing the result of the next roll correctly remain at 1 in 6, however many rolls have been seen previously. Randomness is the characteristic of chance, as illustrated by a fair die.
There have been various attempts to define randomness more formally than this over time, in terms of unpredictability, the non-computability of random events, and the inability to describe a set of random elements more efficiently than by repeating the entire set.
Randomness and social science

Use the code MSPACEQ323 for a 20% discount
The term ‘random’ is widely used in social science in the context of sampling and statistical analysis. A sample is random if all of the cases in it were selected by chance from a larger set of cases known as the population, and if all of the cases in the population had a genuine chance of being in the sample. A population itself is clearly not a random sample, and nor is a sample selected by other means (ad hoc, convenience, purposive etc.). A sample selected at random but in which cases cannot be found, do not respond, or are otherwise not recorded is no longer a random sample. And there is no reason to believe that such missing cases are a random subset of the planned sample. Those refusing to take part in a piece of research, for example, can be predicted on the basis of their prior characteristics with more success than chance alone.
None of the statistical techniques predicated on working with a random sample can or should be used with populations, or with samples that are not random or incomplete. This means that significance tests of significance, p-values, standard errors, or confidence intervals should not be calculated or reported with such cases. It also means that anything like these should be ignored in the work of others unless the study is based on a complete random sample. I have never seen a real study that had a complete random sample, which means that such statistical techniques should rarely, if ever, be reported. However, their abuse remains widespread in the literature.
Real-life samples cannot and should not be used with any technique predicated on random samples. Given that randomness is an ideal not often seen in practice in social science samples, it is most important that the much more common non-random (and incomplete “random”) samples are analysed properly. We need to teach more about the appropriate techniques for handling these real-life samples that are not random, even though these approaches are largely ignored in most statistical texts. What these techniques are will be addressed in future blogs, and are covered in my new book.
More Methodspace Posts about Data Analysis
Dr James Abdey discusses the importance of statistical literacy and applications of methodologies to assist researchers in understanding their data and presenting their results clearly, objectively, and with confidence.
Listen to this interview, and check out Rhys Jones’ latest book: Statistical Literacy: A Beginner's Guide.
Learn about options available in the dynamic landscape of emerging methodological extensions in the PLS-SEM field is the necessary condition analysis (NCA).
Methods Film Fest!
We can read what they write, but what do researchers say? What are they thinking about, what are they exploring, what insights do they share about methodologies, methods, and approaches? In 2023 Methodspace produced 32 videos, and you can find them all in this post!
Christina Silver explains why and how to use qualitative data analysis software to manage and analyze your notes, literature, materials, and data. Sign up for her upcoming (free) symposium!
Use research cases as the basis for individual or team activities that build skills.
How do you find gems in a research project when the data is too thin for generalizations? In this post researchers discuss creative ways to learn from (and write about) the experience.
The focus for Q3 of 2023 was on analyzing and interpreting qualitative and quantitative data. Find all the posts, interviews, and resources here!
Dr. Stephen Gorard defines and explains randomness in a research context.
Mentor in Residence Stephen Gorard explains how researchers can think about predicting results.
The Career and Technical Education (CTE) Equity Framework approach draws high-level insights from this body of work to inform equity in data analysis that can apply to groups of people who may face systemic barriers to CTE participation. Learn more in this two-part post!
The Career and Technical Education (CTE) Equity Framework approach draws high-level insights from this body of work to inform equity in data analysis that can apply to groups of people who may face systemic barriers to CTE participation. This is part 2, find the link to part 1 and previous posts about the Equity Framework.
Instructional tips for teaching quantitative data analysis.
In an era of rampant misinformation and disinformation, what research can you trust? Dr. Stephen Gorard offers guidance!
Learn how inductive and deductive styles of reasoning are used to interpret qualitative research findings.
Images contain information absent in text, and this extra information presents opportunities and challenges. It is an opportunity because one image can document variables with which text sources (newspaper articles, speeches or legislative documents) struggle or on datasets too large to feasibly code manually. Learn how to overcome the challenges.
Tips for dealing with missing data from Dr. Stephen Gorard, author of How to Make Sense of Statistics.
Learn more about standard deviation from a paper and presentation from Dr. Stephen Gorard.
Video capture is ubiquitous. What does it mean for researchers, and how can we analyze such data?
Qualitative data analysis varies by methodology. Learn about approaches for phenomenological studies through this collection of open access articles.
Learn about qualitative data analysis approaches for narrative and diary research in these open access articles.
Ethnography involves the production of highly detailed accounts of how people in a social setting lead their lives, based on systematic and long-term observation of, and discussion with, those within the setting.
Qualitative data analysis varies by methodology. Discover diverse ways to analyze data for grounded theory studies in these open access articles.
Qualitative data analysis varies by methodology. Learn about approaches for action research in these open access articles.
How can you study digital culture and activism? Watch this interview with Dr. Lyndon Wray.
Look at the choices of video methods made by authors of four research articles.
This collection of open-access articles includes qualitative examples of analysis strategies to use with multimedia video data.
This collection of open-access articles includes quantitative examples of analysis for video data.
How do we understand and interpret visual or video data? See these open-access articles for ideas and examples.
Looking back at 2023, find all posts here!
We explored stages of a research project, from concept to publication. In each quarter we focused on one part of the process. In this recap for the year you will find original guest posts, interviews, curated collections of open-access resources, recordings from webinars or roundtable discussions, and instructional resources.